Texture smoothing technologies have undergone significant transformations over the years, evolving from basic algorithms to sophisticated machine learning innovations. This evolution has not only enhanced the quality of images but has also revolutionized various fields, including medical imaging, graphic design, and video production.
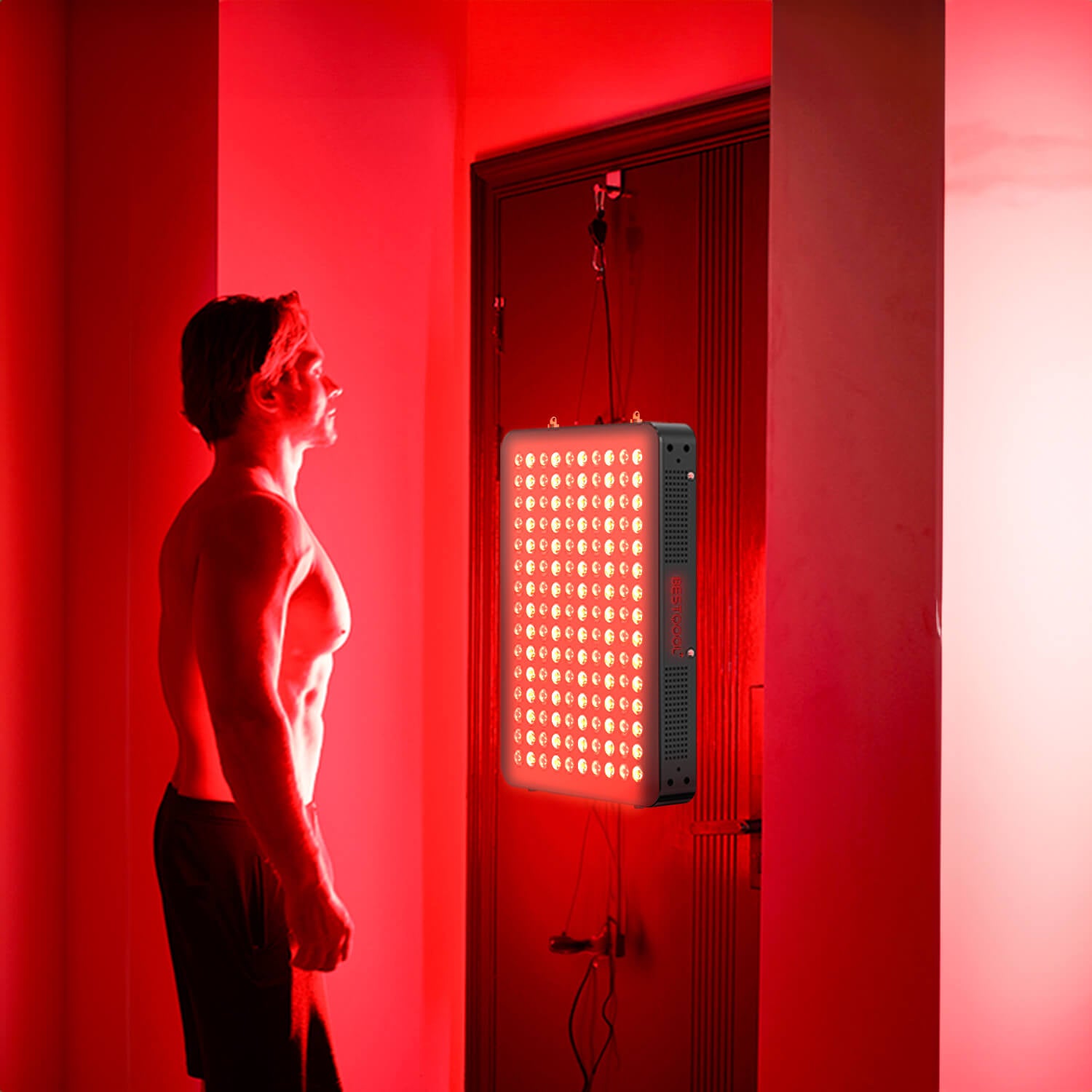
Understanding Texture Smoothing Technologies
At its core, texture smoothing technologies aim to reduce noise and enhance the visual quality of images. Early methods primarily relied on linear filtering techniques, which often resulted in a loss of important details. As technology advanced, more complex algorithms emerged, allowing for better preservation of edges while smoothing out unwanted textures.
Early Algorithms: The Foundation of Texture Smoothing
In the early days, texture smoothing was achieved through simple algorithms such as Gaussian and median filters. These methods were effective but had limitations. For instance, Gaussian filters could blur edges, while median filters were computationally intensive. As a result, researchers began exploring more advanced techniques.
- Gaussian Filters: Used for blurring images, but often at the cost of edge detail.
- Median Filters: Effective in removing noise but computationally demanding.
The Rise of Non-Linear Techniques
As the demand for high-quality images grew, non-linear techniques gained popularity. These methods, such as bilateral filtering, allowed for better edge preservation while smoothing textures. They work by considering both the spatial distance and intensity difference between pixels, leading to more refined results. Have you ever wondered how these techniques manage to maintain detail while reducing noise? The answer lies in their ability to adaptively smooth based on local image characteristics.
Machine Learning Innovations in Texture Smoothing
Today, the advent of machine learning has transformed texture smoothing technologies once again. Deep learning models, particularly convolutional neural networks (CNNs), have shown remarkable success in this domain. These models can learn complex patterns and features from large datasets, enabling them to perform texture smoothing with unprecedented accuracy.
- Convolutional Neural Networks (CNNs): These networks excel at recognizing patterns and can effectively smooth textures while preserving critical details.
- Generative Adversarial Networks (GANs): GANs can generate high-quality images, making them useful for enhancing texture smoothing processes.
Future Directions for Texture Smoothing Technologies
The future of texture smoothing technologies looks promising. As machine learning continues to evolve, we can expect even more refined techniques that will push the boundaries of image quality. Researchers are exploring ways to integrate real-time processing capabilities, making these technologies accessible for various applications, from mobile devices to high-end medical imaging systems.
In conclusion, the journey of texture smoothing technologies from early algorithms to modern machine learning innovations highlights the importance of continuous improvement in image processing. As we move forward, embracing these advancements will undoubtedly lead to enhanced visual experiences across multiple domains.